Where is the AI? A Look into the Field of Automatic Prostate Cancer Detection and the Role of Data
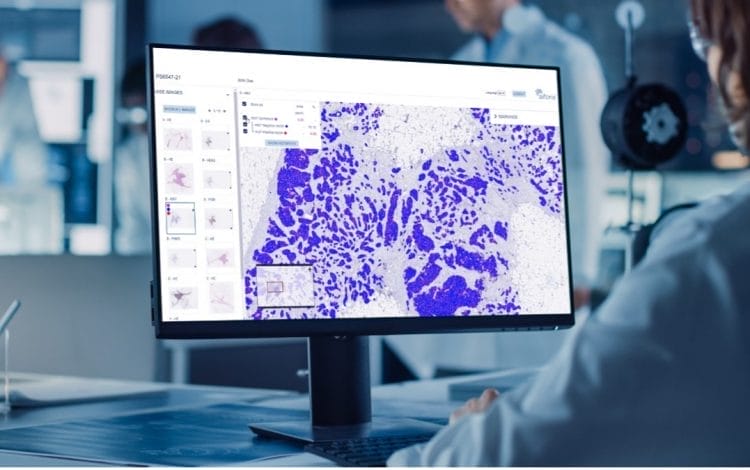
Ever wondered if one day you could be treated by an AI doctor or receive a diagnosis from an algorithm? Frewing et al. examines the potential of artificial intelligence in the field of prostate pathology in a recent systematic literature review.
Image recognition advancements in the field of artificial intelligence are poised to radically reshape the field of medical imaging. This is why we developed our life science image hub module for our platform. Over the last five years, a multitude of researchers and institutions have embarked on a captivating journey: the creation of an artificial intelligence algorithm capable of autonomously detecting and classifying prostate cancer from biopsy samples. This technological feat holds the promise of liberating pathologists from the mundane aspects of their work, granting them more time to tackle the intricate challenges that define their profession. Enter the realm of algorithms, where innovation converges with medical precision.
The Algorithmic Challenge: Analyzing Hundreds of Tissue Samples
On any given day, a pathologist is usually tasked with reviewing hundreds of tissue samples, all of which they must comb through carefully and meticulously to note for cancerous bodies. Making the slightest error of omission could have extremely tragic consequences, which is why researchers have been working to develop an algorithm capable of detecting cancer in these samples. Ideally, this algorithm would be able to process tissue samples with lightning speed and properly annotate all the glands in the image. If trained properly this algorithm could serve as a secondary expert opinion for any anomalous or suspicious cases.
Navigating the Gleason Grading System: Beyond Binary Distinctions
Efforts to develop this extraordinary tool have recently stalled. Preliminary algorithms mainly focused on distinguishing benign tissue from cancerous tissue, for the most part have been partly successful in achieving this outcome. Making the distinction between benign tissue and potentially cancerous tissue is unfortunately of little value because cancerous tissue is not binary but exists on a spectrum. This spectrum in prostate cancer, is known as the Gleason grading system, which rates the severity of the cancer on a scale of 1 to 5 with each number determining critical diagnostic and prognostic decisions. Training an algorithm that can replicate a pathologist’s ability to sort glandular tissue according to the Gleason grading system is of immense value but requires algorithms to move beyond the binary distinction of benign and cancerous tissue and on to a more nuanced multiclassification scale.
Figure 1 shows how multiclassification tasks across the spectrum of literature were consistently less accurate on average when compared to binary tasks. Accuracy measurements included standard accuracy, area under the curve (AUC), and kappa values for comparison.
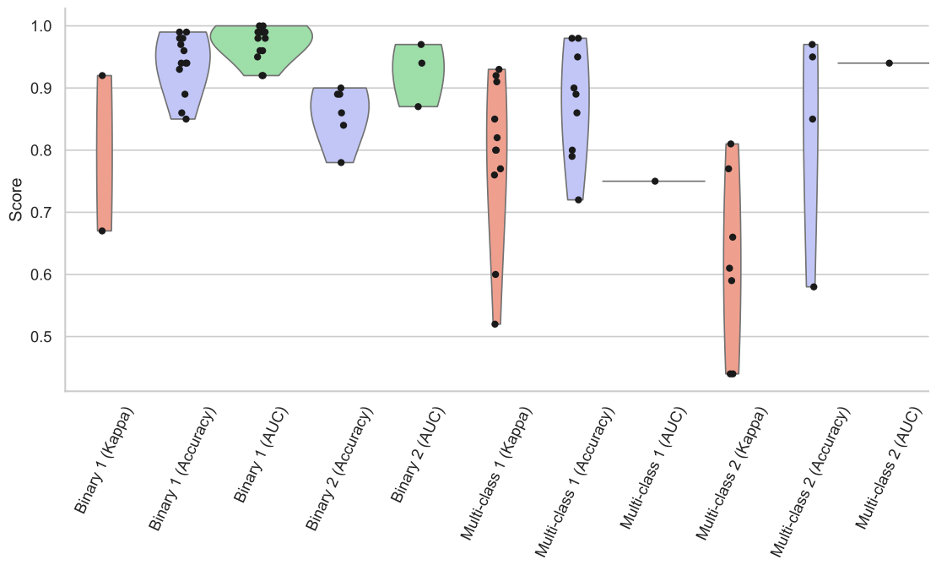
In the same review, the researchers examined the clinical applicability of existing developed algorithms. One of the biggest roadblocks to clinical relevance was the generalizability of the developed algorithm. Most of the developed algorithms did not attempt any form of clinical testing, and even from the small group that did attempt clinical testing only one showed decisive results.
Poor generalizability stems directly from the lack of quality data from which algorithms could be trained. This unfortunately requires a large corpus of data which oftentimes is difficult to access due to privacy laws and restrictions on health-related data. Data which accurately represents what is observed in clinical practice along with annotations from a variety of pathologists would yield the best representation for training.
Hart & Engler et. al. sampled data from the largest public repository of prostate cancer biopsies and trained an algorithm to classify the cancerous tissue according to the Gleason grading system. This preliminary study found that the concurrence between the algorithm and an expert pathologist to be minimal. The researchers concluded that the existing publicly available data was insufficient to train a high-performance cancer detection tool. AI researchers have long understood the wisdom of the “garbage in garbage out” principle, which refers to the fact that an algorithm trained on poor data will generally have poor overall performance. In Figure 2, researchers compiled a set of critically important factors in the creation of an ideal data set specifically targeted for prostate cancer. Although some of the specifications are in regard to prostate cancer the overall principle they convey would be applicable to most medical imaging AI tools.
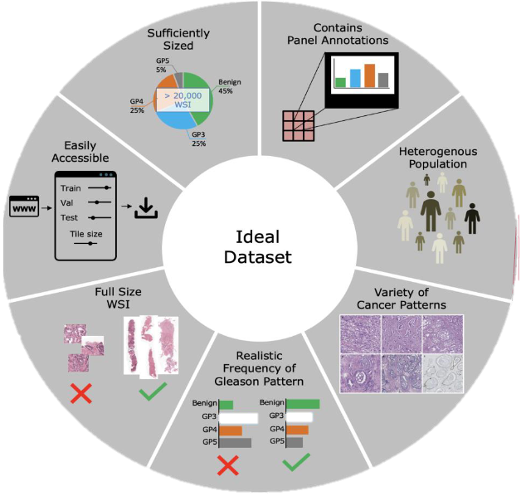
In conclusion, if the goal of researchers is to develop a functionally useful AI tool, more time may have to be spent curating and preparing a clinically representative data set. Currently, the approach by researchers seems to be to test out new architectures on the same data hoping that a modified architecture will make a breakthrough and achieve optimal performance with minimal training. This makes effectively managing with a LIMS consolidation platform and backing it up with to a life science data preservation platform essential. While throwing modified architectures at the problem may eventually result in a more effective tool, data may prove to make a bigger impact.
Sources: