Making Chemistry Knowledge Machine-Actionable
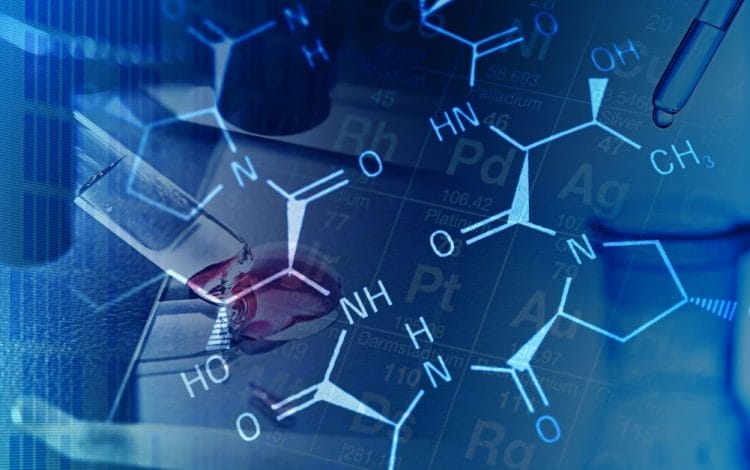
The history of chemistry has been epitomized by individual chemists coming up with hypotheses, running experiments at lab-scale, and producing discoveries. But in 2022, chemistry data is generated at a scale previously unseen, computers can rapidly process that data, and the data can be widely distributed at relatively minimal cost.
This new frontier of global-scale chemistry research offers new opportunities for scientific discovery, but requires new infrastructure to enable those discoveries. Jablonka et. al. published a Nature article detailing the current state of chemical data management systems and best practices. Here we condense this article into actionable takeaways.
Data management can be segmented into three tasks: data collection, data processing, and data publication.
Data Collection
The key to data collection is making the data standardized and inter-operable. There are two ways to do this: standardization at the source, and standardization upon entry in an electronic lab notebook (ELN). It is desirable for standardization to occur from the source instrument. When this is not possible, the authors argue that the most important function of an ELN is to convert the data into a standard, inter-operable format.
These are only a sampling of chemistry file formats. For more, check out this list.
If standardization occurs upon entry to an ELN, care must be taken to avoid undue burden for the researcher. Manual conversion of a large mass-spec dataset is a non-starter. Instead, a hybrid manual approach for smaller experiments seems best. One approach to this is a template format for text entry, as is used in some ELNs. For example, “…we added R1 (xR1 g), R2 (xR2 g), R3 (xR3 g), in a y%R4/ (100–y)%R5 mixture and put the solution in oven y for t h at T °C…”. A template approach requires minimal additional overhead to document the procedure, but yields data that will be much more useful in the future.
Data collection is also an important aspect of ZONTAL’s mission. See our recent reports to understand how we harmonize data: https://doi.org/10.1016/j.drudis.2021.07.019 , https://link.springer.com/chapter/10.1007/978-3-030-89906-6_62
Data Processing
Once data is in a standard format, it becomes “machine-actionable”. Computation on standardized data can have many purposes:
- Search and indexing: Making data searchable is the first step towards making it accessible.
- Linking to ontologies: Linking data to existing ontologies can yield new insights. For example, by linking mass spectrometry data to a database of mass spectrometer manufacturing data might reveal trends in measurements by manufacture date.
- Linking to online APIs: There are a plethora of tools for computational chemistry analysis. Ideally, an ELN would allow you to make API calls and incorporate the results in your ELN. For example, you may want to compute the expected X-ray diffraction pattern for a crystal structure and compare it with your measurements.
- Machine learning: Standardized data is essential for machine learning. Machine learning models trained on large-scale chemical data can yield insights that are not apparent from manual analysis.
Caption: Several examples of data processing that can be done on lab notebook data.
Data Publication
Finally, data must be published in a useful way. In an age where data is not limited to what can be printed in a physical journal, it would be useful for readers to be able to interact with the raw data used to create figures. This involves incorporating data import/export capabilities into ELNs, a feature missing from many ELNs. This is why we developed our ELN archiving solution so that data can be backed up from various ELNs and securely stored for future reference and use.
Another important aspect of publication is making data permanently accessible. For this purpose, digital object identifiers (DOIs) create permanent identifiers by which data can be found.
Conclusion
Benefits of making data machine actionable:
- Allows for data search
- Allows you to link with ontologies
- Allows you to link with online APIs that process data and yield new insights
- Allows machine learning on data, especially by including “failed” experiments
- More transparent and actionable experience for paper readers
- Better tracking of data provenance
- Allows automated error-checking of data
- Data is IP and has value. The more accessible the data, the more valuable it is
Tools to start using:
- ELNs
- Instruments with automated data digitization, including data provenance metadata
- Labforward
- Metadata describing data provenance
- Including Bioschemas and Material Schema metadata markup on web resources – https://bioschemas.org/ https://pages.nist.gov/material-schema/
- Use existing file formats and export tools, like Zenodo
- Use stable, unique identifiers (DOIs)
Tools to create:
- Conversion tools for ELNs – convert incoming data to an interoperable format
- ELN export tools, allowing for easy publication/dissemination
- New standards. Which areas are missing standards? What problems would be solved by introducing standardization in this area? Is there an existing standard that you can build on?
Trade-offs to consider:
- Standards – improve existing standards vs. making new ones
- Convenience for researchers vs. accessibility for machines
If this still seems like a lot to manage, consider hiring a company that specializes in lab digitization and automation – like us! Feel free to explore our website or contact us here.