Data Centricity: Key for the Successful Digital Journey Towards a Digital Lab
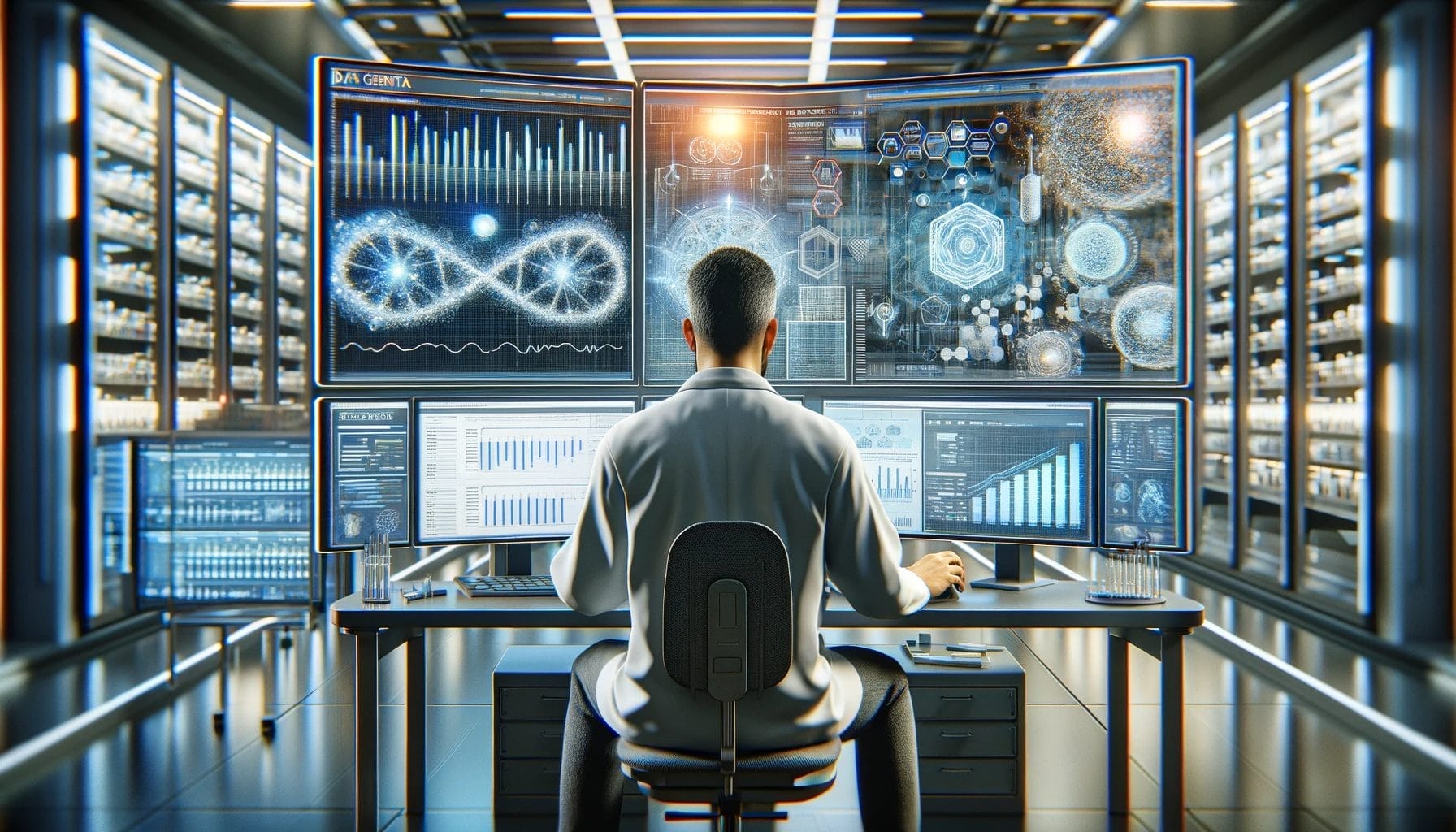
While data meshes and data fabrics are often discussed, data centricity is frequently not consequently executed, despite its significant implications. Data centricity places data at the core of operations and decision-making processes, going beyond mere data utilization in applications.
To implement data centricity, three principles are crucial: recognizing data as a key asset, ensuring data is self-describing, and using open, non-proprietary formats.
In practice, data centricity is the overarching bracket of data mesh and data product concepts. Simplified: A data mesh is a decentralized approach to data architecture, enabling domain-specific teams to manage their data autonomously and at scale. A data product is an instance of a data mesh architecture that generates business value, while a data platform serves as an enabler for data centricity and may execute the data mesh concept.
Now, what do the principles mean in reality? In the laboratory environment, these principles come to life:
Recognizing Data as a Key Asset
Every one of us agrees with this, sure, but what are the implications of this principle?!
Let’s consider simple examples: A chemical structure, a new modality, an experiment, a study, or a pharmaceutical or medical product. If we simply look at the terminologies, terms and data concepts are often still understood differently from function to function, in projects, or in collaborations, which causes losses in time and ties up resources.
In the lab, scientists are redoing experiments because they don’t trust the old data. The reason is that the “old” data from 5 years ago is not well described and not traceable. So, what we need in addition to the compound are all contextual metadata, operational data across the processes, and data life cycles.
New compounds in living test systems show biological reactions or molecular interactions, but they come with a high level of variability. When scientists plan a new study, they may find the individual results of old experiments not representative of the current conditions or questions they are addressing, making it seem unreliable for reuse. A biologist should not only look at individual experiments from the past and decide if to re-run them but instead look more holistically at the legacy data for patterns that might help answer questions for the next project, almost like asking multiple senior lab scientists for their advice.
Patterns in legacy data might only be visible once the old experiment results, together with their metadata and descriptions, are made FAIR. Only then do statistical analyses, visualization techniques, and data mining uncover relationships that may not be apparent when examining individual assay results in isolation. A new understanding, also from failed experiments, helps in automation processes because it narrows down the degrees of freedom during the design phase.
Ensuring Data is Self-Describing
What does self-describing mean? Let’s re-engage with the compound that is being tested in many experiments across its whole life cycle. You want to know, when it has been tested, which results, under which conditions, with which requested parameters, the method and the business context, and the overall workflows.
If we collect all information in interconnected data packages, including all available operational and process data, solutions will change from application-centricity to data-centricity. A LIMS or ELN will look different in the future. It will be more of a portfolio of application services than an application.
A data platform plays a crucial role in enabling data centricity. It encompasses the end-to-end data lifecycle, operational efficiency from FAIR data principles to business process automation, and life science analytics from descriptive data science to prescriptive analytics. This enables customers to develop their own AI models using their data assets.
Choosing the right platform that considers these aspects and is vendor-agnostic is essential. The mantra is to have full control of your data in a fully self-describing way—flexible and configurable.
Using Open, Non-Proprietary Formats is Crucial in Today’s Digital Landscape
Similar to the impact of MP3, the de facto open standard in the music industry, open standards play a significant role. The Allotrope Foundation has developed the Allotrope Data Format (ADF) and the Allotrope Simple Model (ASM), which serve as scientific data standards for laboratories. Additionally, the ISA 88 standard is essential for batch processing, while the ISO OAIS reference architecture ensures long-term data preservation and interoperability with other systems through an open API architecture. Considering these factors when selecting technology or solutions is important.
The Digital Lab: About Vision and Maturity
Experiments and studies generate data that can span from minutes to years, all related to specific products such as substances or biological entities. To realize the value of these data products for patients and researchers, the data and data integration architecture must support their long lifecycles.
Embracing data centricity empowers digital labs to unlock the full potential of their data, drive innovation, and make informed decisions. It ensures that data takes center stage in operations, enabling organizations to thrive in the digital era.
Where are you on your digital journey in the lab landscape? In your transition from FAIR data to automated tests,? In your journey from data centricity to business centricity,? And in your life sciences analytics, how are you progressing from descriptive data analytics to prescriptive data analytics? We are happy to provide a maturity assessment of your digital lab journey. In any case, we encourage you to save costs, time, and energy by gaining more insights into what works and what doesn’t.