It seems we can’t find what you’re looking for
Featured Resources
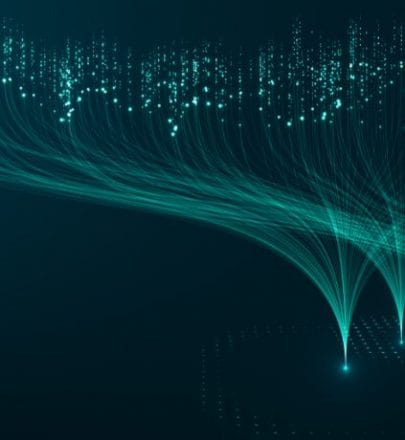
White Paper
From Bottlenecks to Breakthroughs: Scaling Scientific Data Processing from On-Prem to Cloud for AI-Ready Pharma
Article
Unlock the Power of Your Data: ZONTAL’s New Analytics Offerings
White Paper
Achieving AI Readiness in Scientific Research: Leveraging ZONTAL for Data Standardization and Sustainability