AlphaFold Rises to New Heights with Version 3
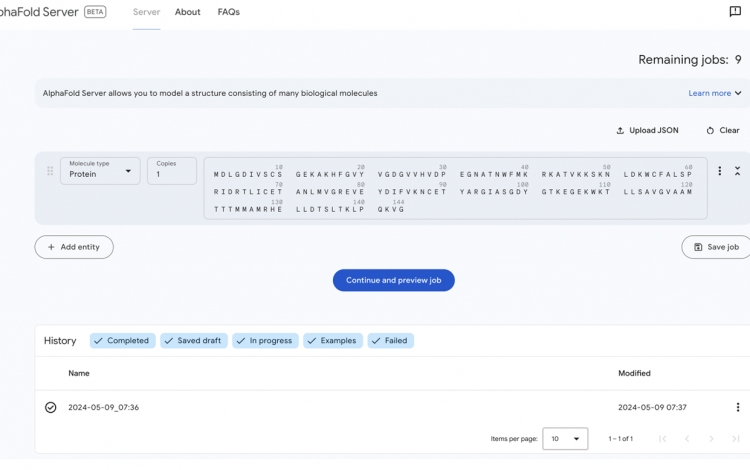
In the ever-evolving landscape of computational biology, the AlphaFold team at DeepMind has once again pushed the boundaries with the introduction of AlphaFold 3 (AF3). This latest iteration represents a significant leap forward, offering unparalleled accuracy in predicting the structures of complexes spanning nearly the entire molecular spectrum found in the Protein Data Bank (PDB).
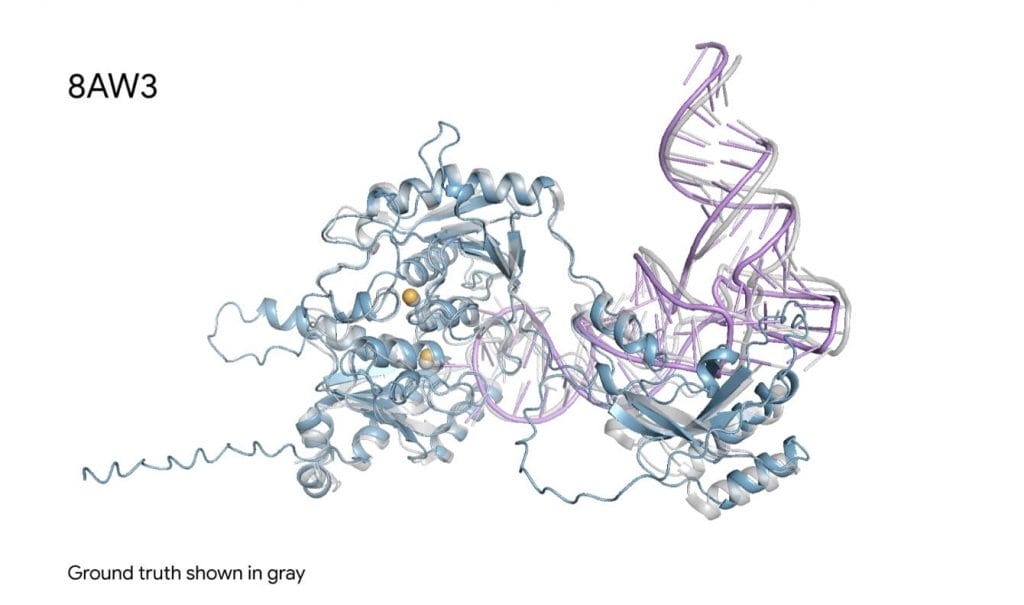
Evolutionary Leap from AlphaFold 2
While building upon the foundation laid by its predecessor, AlphaFold 2, AF3 boasts several key advancements that set it apart. At its core, the architectural changes are driven by the need to accommodate a wide range of chemical entities without excessive complexity.
One of the most notable modifications lies in the replacement of the Evoformer with the simpler Pairformer Module, significantly reducing the amount of multiple sequence alignment (MSA) processing required. Additionally, AF3 directly predicts raw atom coordinates through a Diffusion Module, eliminating the need for amino-acid-specific frames and side-chain torsion angles used in AlphaFold 2.
Another innovative aspect of AF3 is its ability to handle molecular complexity with elegance. The multiscale nature of the diffusion process allows the model to accommodate arbitrary chemical components without the need for explicit stereochemical losses or extensive special-casing of bonding patterns.
In line with some recent work, the researchers found that no explicit invariance or equivariance with respect to global rotations and translations of the molecule is required in the architecture. By omitting these components, they were able to simplify the machine learning architecture further.
One of the key challenges in generative models is the tendency to hallucinate plausible-looking structures in unstructured regions. To counteract this effect, the researchers employed a novel cross-distillation method, enriching the training data with AlphaFold-Multimer v2 predicted structures. In these structures, unstructured regions are typically represented by long extended loops instead of compact structures, “teaching” AlphaFold 3 to mimic this behavior and reducing hallucination.
Unparalleled Performance and Capabilities
AlphaFold 3’s advancements translate into remarkable performance metrics. Across various categories, it achieves significantly higher accuracy than specialized methods tailored for specific tasks, including protein structure prediction and modeling protein-protein interactions.
One of the most impressive feats of AF3 is its blind docking capabilities. The model can predict structures from input polymer sequences, residue modifications, and ligand SMILES strings, outperforming classical docking tools like Vina and other blind docking methods by a substantial margin.
Moreover, AF3 exhibits exceptional accuracy in predicting covalent modifications, such as bonded ligands, glycosylation, and modified protein residues and nucleic acid bases. This capability holds immense potential for understanding the intricate details of biomolecular systems.
Looking Ahead: Cellular Component Modeling
As the authors of the AlphaFold 3 paper suggest, the development of bottom-up modeling of cellular components is a critical step toward unraveling the complexity of molecular regulation within the cell. The remarkable performance of AF3 demonstrates that developing the right deep learning frameworks can significantly reduce the amount of data required to obtain biologically relevant performance on these tasks, amplifying the impact of the data already collected.
With AlphaFold 3, the DeepMind team has once again raised the bar for computational biology, paving the way for further advancements and discoveries in this rapidly evolving field.
Testing the Tool: Predict a CASP16 Target
Unfortunately, Google once more opted to not release the AF3 code base. In the past, this has not stopped the scientific community to train similar models in the wild (https://www.biorxiv.org/content/10.1101/830273v1). With CASP16 currently ongoing, we are likely going to read about further method advancements by the end of the year. It’s a race at an incredible pace, let’s look forward to many new drugs entering the market thanks to these advancements!
Luckily, using the google server you can fold proteins, multimers, and ligands at: https://alphafoldserver.com/. The main issue is that only 10 jobs token are awarded per day, to generate the thousands of structures that you want to rank from a diffusion-based model makes this server more a marketing strategy than the research tool the community is longing for.
But let’s go and give it a test, and run the first CASP16 monomer target through the server. The sequence information comes from: https://predictioncenter.org/casp16/target.cgi?id=61&view=regular
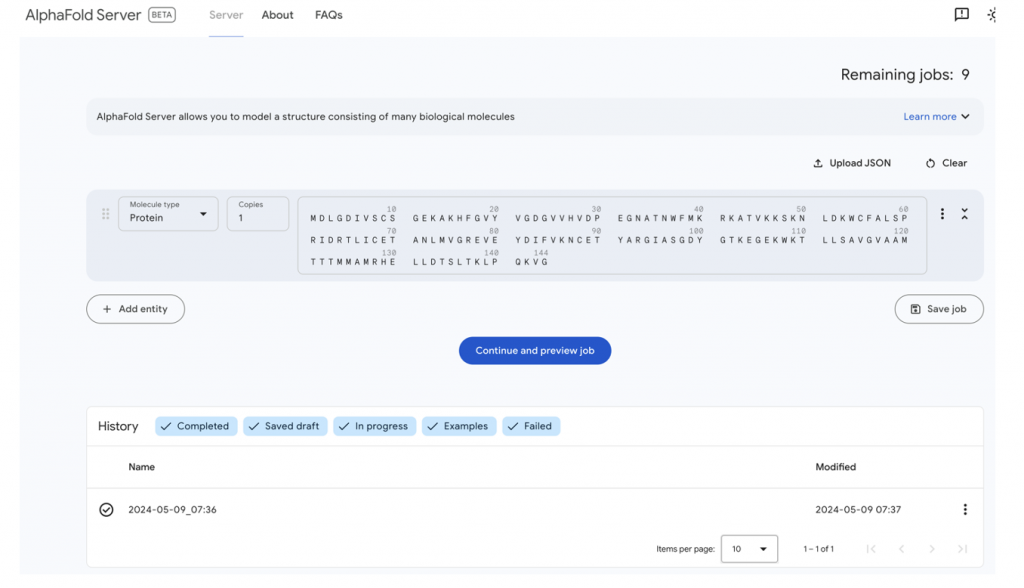
Only a few minutes later, the job results are available.
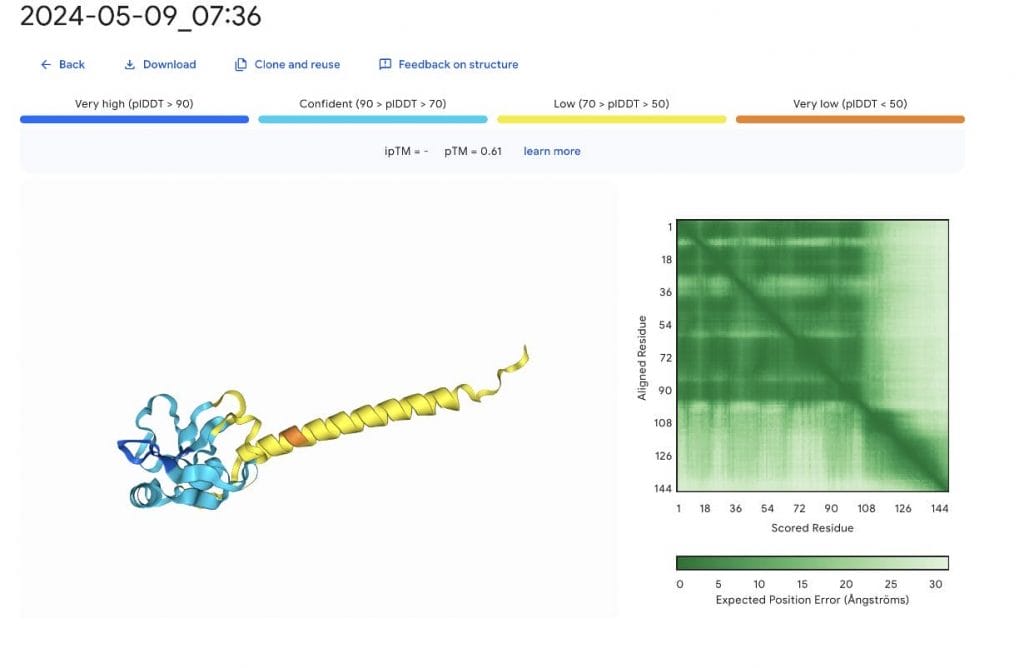
The likely disordered region in yellow is problematic. Here, I wish for an ensemble of structure to select from. Not wanting to waste tokens, we repeat the prediction with a different number seed.
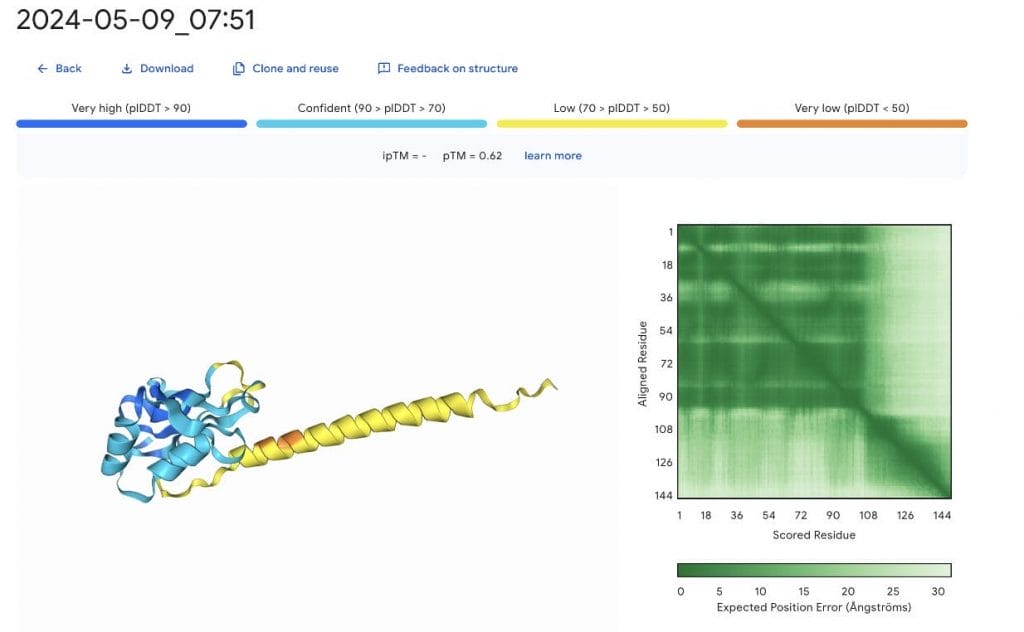
Most likely the very low confidence region in orange could cause the extended helix to reorient within solution. This reveals the great limitation of all structure prediction models up to date, they try to reproduce crystal structures and not physiological behavior. But worthy of a Nobel are these advancements still!