Unlocking the Power of Scientific Data: A Data Scientist’s Perspective
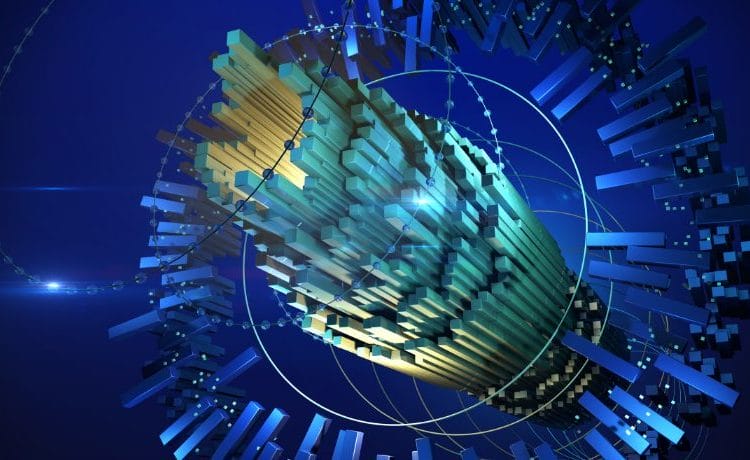
We data scientists are familiar with the vast sea of information that surrounds us. Whether the goal is to analyze customer behavior, predict market trends, or fine-tune logistics processes, data serves as the guiding force in every endeavor. Yet beyond the usual sources lies a relatively untapped goldmine – scientific data. This data holds immense potential for innovation, when properly harnessed.
The Challenge of Managing Scientific Data
Scientific research produces immense amounts of data, often fragmented across different platforms, formats, and institutions. This presents a challenge: how can researchers, labs, and institutions effectively manage and utilize this data? This is where Scientific Data Management Systems (SDMS) come into play.
Much like a Swiss Army knife, an SDMS offers scientific companies a solution for storing, organizing, and safeguarding research data. The true value lies in its ability to help researchers navigate the data-landscape of scientific findings. From massive data sets to the nuances of single experiment results, an SDMS is the backbone that supports both individual projects and larger research initiatives.
Why It Matters: Making Data Work for Science
A well-designed SDMS provides more than just a repository for data; it transforms how researchers interact with and collaborate on their findings. By enhancing findability, it eliminates the hours spent sifting through disorganized files, poorly labeled data, and waiting for a co-worker to share their results from a year ago. Proper metadata and standardized identifiers ensure that every piece of information is put into a catalog and made accessible when needed. It’s like turning research notes into a fine-tuned search engine.
But there’s more: accessibility ensures that data is not trapped in siloed systems. Instead, it is readily available to researchers, data scientists, and AI (Artificial Intelligence) systems that rely on it for further exploration. An SDMS fosters collaboration where findings can be shared and built upon by a broader scientific community.
Then there is the matter of interoperability – an SDMS communicates across various platforms, databases, and lab instruments. It breaks down barriers between disparate systems and enables data exchange. Think of it as a translator between different scientific “languages” allowing integration of complex experiment data with computational models.
Finally, we come to reusability. Scientific research is an iterative process where each discovery stands on the shoulders of previous work. A robust SDMS preserves valuable data for future generations, allowing others to extend research further.
A Guiding Framework: The FAIR Principles
The FAIR principles – Findable, Accessible, Interoperable, and Reusable – act as guiding tenets for managing scientific data. An SDMS that adheres to these principles helps ensure that data is not only useful today but remains a valuable resource for future scientific exploration.
By ensuring that data is findable through proper structuring and metadata, it becomes a tool that can be leveraged quickly. Accessibility makes it open to the scientific community, fostering collaboration across borders and disciplines. When data is interoperable, it allows different systems and formats to work together without conflict. And by being reusable, data continues to support research long after the original study has concluded, propelling innovation forward.
The Role of Ontology: Bringing Meaning to Data
Ontologies, though often seen as abstract concepts, play a crucial role in making scientific data more comprehensible and usable. Simply put, an ontology is a structured framework that provides context and relationships between different data points. It allows a level of understanding and categorization that goes beyond simple labels and keywords.
By assigning meaning to the data, ontologies enable more sophisticated data analysis and integration. They help ensure that terms like “experiment” or “molecule” are universally understood in their scientific context, avoiding the ambiguities that can hinder research.
Additionally, ontologies support quality assurance, helping to maintain the integrity of data sets. When erroneous or impossible entries – such as mythical creatures like unicorns – are introduced, the ontology provides a safeguard by raising flags that something might be wrong.
Embrace the Future of Scientific Discovery
For today’s data scientists, engaging with scientific data can open a world of possibilities. With the right tools – like an SDMS and robust ontologies – navigating the complex landscape of scientific research becomes more efficient and rewarding. These tools not only enhance data management but also help unlock deeper insights, pushing the boundaries of knowledge.
Whether you’re trying to uncover new drug modalities or to explore biological processes, the ability to organize and leverage scientific data is an invaluable asset. Embrace the tools and principles that will guide your data-driven journey into uncharted scientific territories.
Laying the Foundation for AI and LLM (Large Language Models) Use Cases
The application of Artificial Intelligence (AI) and Large Language Models (LLMs) in data science is becoming more prevalent every day. However, their effectiveness depends on the quality and structure of the data they are trained on. This is where FAIR data within an SDMS system, enriched by ontologies, becomes indispensable. By ensuring data is findable, accessible, interoperable, and reusable, SDMS provides a solid foundation for AI and LLM models to interpret and utilize complex datasets efficiently. Ontologies play a crucial role in giving context and semantic structure to the data, enabling AI models to process information with greater accuracy. Whether it’s training AI algorithms for scientific discovery or deploying LLMs to analyze vast research databases, FAIR data ensures that AI and machine learning can operate at their full potential, driving innovation and uncovering new insights across a range of data science applications.
ZONTAL is Transforming Scientific Data for AI Integration
For organizations who would like to harness the full potential of their scientific data, ZONTAL offers an innovative solution that integrates analytics and data science into everyday laboratory workflows. By embedding FAIR principles in every processing step of the data, ZONTAL transforms complex datasets into accessible, interoperable, and reusable formats, ensuring scientific data is well-organized and ready for advanced analysis. Beyond simple data management, ZONTAL creates a vendor-neutral environment, eliminating siloed systems and offering instant access to actionable insights across instruments, workgroups, and between collaboration partners. By bridging the gap between raw data, AI, and machine learning applications, ZONTAL is the platform where large language models can be plugged-in, enabling future proof data-driven decision-making for scientists.
Further Reading:
- Scientific Data Cleansing and Data Management for Artificial Intelligence (AI)
- Beyond Traditional AI: Embracing Multimodal Challenges with Meta-Transformer
- LoRA: An Efficient Way to Finetune Giant Language Models
Ready to harness the power of your scientific data?
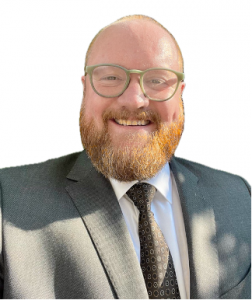