Reality or Illusion – What can AI do for Drug Discovery?
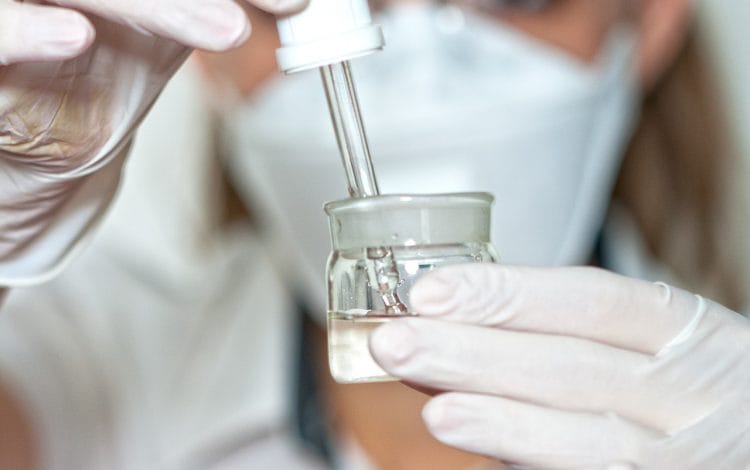
I thoroughly enjoyed meeting Andreas Bender at the recent BioTechX conference in Basel. He gave a very honest and thought-provoking presentation on a series of papers released in Drug Discovery Today, titled: Artificial intelligence in drug discovery: what is realistic, what are illusions? Let’s recap his main findings:
Artificial intelligence (AI) has had a profound impact on many areas of research and technology, particularly in image recognition and natural language processing. However, comparable advances in drug discovery are relatively rare. This article examines the stages of drug discovery and quantifies the impact that improvements in the time taken, success rate, or affordability can have on bringing new drugs to market.
One of the main challenges in drug discovery is the high failure rate of clinical trials. In the early stages of drug discovery, the success rate is typically low, with only a small percentage of compounds progressing from pre-clinical to clinical trials. The clinical success rate is also relatively low, with only a small percentage of compounds that enter clinical trials receiving regulatory approval.
Figure 1 Impact of 20% improved speed, quality, or cost in million USD on the various stages of drug discovery (adapted from Bender et al.).
Changes in clinical success rates have the most profound impact on improving success in drug discovery (see Figure 1). In other words, the quality of decisions regarding which compound to take forward (and how to conduct clinical trials) is more important than speed or cost. Current advances in AI focus on how to make a given compound, but the question of which compound to make, using clinical efficacy and safety-related end points, has received significantly less attention.
As a result, current proxy measures and available data cannot fully utilize the potential of AI in drug discovery, particularly when it comes to drug efficacy and safety in vivo. Therefore, addressing the questions of which data to generate and which end points to model will be key to improving clinically relevant decision-making in the future.
AI has the potential to revolutionize drug discovery and improve the efficiency of the drug development process. By using AI to analyze large amounts of data and make predictions, researchers can identify potential drug candidates more quickly and with greater accuracy. This can help reduce the time and cost of drug development, and ultimately lead to new and effective treatments for a range of diseases.
However, significant challenges remain, including the need for high-quality data and the development of robust algorithms that can make clinically relevant predictions. Many of the data sources currently used in drug discovery are fragmented and incomplete, making it difficult to accurately model drug efficacy and safety. Additionally, there is a lack of standardization in the data generated during drug development, which can hinder the development of effective AI algorithms.
Overcoming these challenges will require collaboration between researchers in a range of disciplines, including computer science, biology, and medicine. By working together, these researchers can develop AI algorithms that are specifically designed for drug discovery and can effectively incorporate the diverse and complex data sources used in this field.
In conclusion, while AI has already had a profound impact on many areas of research and technology, its potential in drug discovery is only beginning to be realized. Improving clinical success rates and addressing the challenges of data quality and algorithm development will be key to unlocking the full potential of AI in this important field. With continued research and collaboration, AI has the potential to revolutionize drug discovery and improve the lives of people around the world.