Scientific Data Cleansing and Data Management for Artificial Intelligence (AI)
The world of science is coming to a pivotal point in its existence, and it concerns proper scientific data generation, management, and, ultimately, reuse. The FAIR (Findable, Accessible, Interoperable, and Reusable) principles are an example of assessing a healthy scientific data environment. They are also a basis for producing Model-Quality data, which is needed to fuel the AI/ML today and in the future. The more tools we use to gain scientific insight and understanding the sooner we can understand and solve complex biology and chemistry problems or unknowns.
In case you haven’t noticed, some serious theoretical and belief discussions are currently happening regarding the approach to scientific generation and its automated level of curation and reuse within the life sciences industry. Camp 1 says, generate the data and don’t worry about the metadata we can add it later, maybe even with AI. Camp 2 says whoa, you must capture all the necessary metadata (and more) at the time of data collection to produce Model-Quality Data. Any follow-up imputation will be inaccurate, impact data quality, and introduce bias.
Proponents of automated data cleansing believe that tools and algorithms will automatically identify data issues (duplicates, errors, type, etc.) and inconsistencies and correct or update them, thus improving scientific data management accuracy and quality. We don’t think anyone will argue with this except for the possibility that it can be done even better at the time of collection.
Proponents of manual data cleansing processes, especially in smaller organizations or specific industries, might believe that human involvement or oversight is invaluable in understanding the data context and making informed or rule-based decisions about data quality.
There is also a case for a mixed manual-automated data cleansing world. Maybe specific data workflows or use cases get promoted to automation after a certain period of maturity or evolution.
Model-Quality data advocates believe that it is more important to produce high-quality data from the get-go and focus more on quality than quantity. As the phrase states, model quality ensures that scientific data is ready to be used in machine learning (ML) and AI due to rigorous data validation techniques, continuous monitoring, and adopting best practices for data governance.
One con to data cleansing, imputation, and change is the ethical implication of “changing” or altering data. The reality of adding bias, whether accidently or even with intent, can dramatically impact scientific data quality or value. If you consider it, the practice can undermine ML or AI and prevent higher efficiency in drug or therapy discovery or clinical outcomes.
There is such a thing as big data . Examples of some big data are multi-omics data, imaging data, clinical data, etc. These data are very costly to produce and even more complicated to integrate; typically due to poor contextualization/metadata capture. Groups focusing on the challenges of managing large datasets from various sources will advocate for strategies that enable seamless integration and cleansing of data across multiple platforms and formats while ensuring consistency and usability for AI applications. Besides proper metadata, this challenge requires high-quality DOE (design of experiments), data standards, and curation/management. Examples might include versioning of data and algorithms, summarization rules, etc., to achieve proper curation and management.
At the end of the day, the sooner organizations can achieve functional Data Governance and Compliance through an adoptable Scientific Data Strategy, the better off they are from a data quality, data integration, and collaboration perspective.
Each approach presents valuable insights, and the best approach often depends on the specific context and requirements of the AI project in question.
What a coincidence that AI is in the Acronym F.A.I.R.
ZONTAL is at the forefront of creating and managing FAIR data environments for life sciences. Their platform focuses on these principles to achieve Model-Quality data and ensure that complex scientific data environments can integrate when needed to maximize insights and discovery.
Future Trends:
- Ethical Data Practices: There is an increasing awareness of the moral implications of data usage in AI. Organizations focus on ethical data management practices to safeguard privacy and promote trust in AI technologies.
- AI-driven Data Management: The use of AI and machine learning in data management processes is on the rise. These technologies can help automate various tasks and provide insights into data quality and integrity.
In summary, the focus on data cleansing and management is shifting towards automation, ethical considerations, and continuous improvement as organizations recognize the critical role these factors play in the successful deployment of AI solutions and systems.
Discover how ZONTAL helps unlock the potential of AI-powered insights in life sciences.
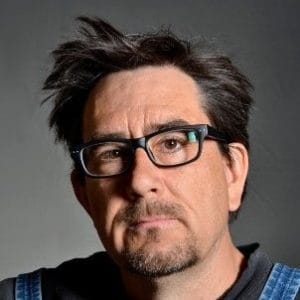