Unlocking Nature’s Molecular Secrets: Introduction to How AI is Transforming Metabolome Research
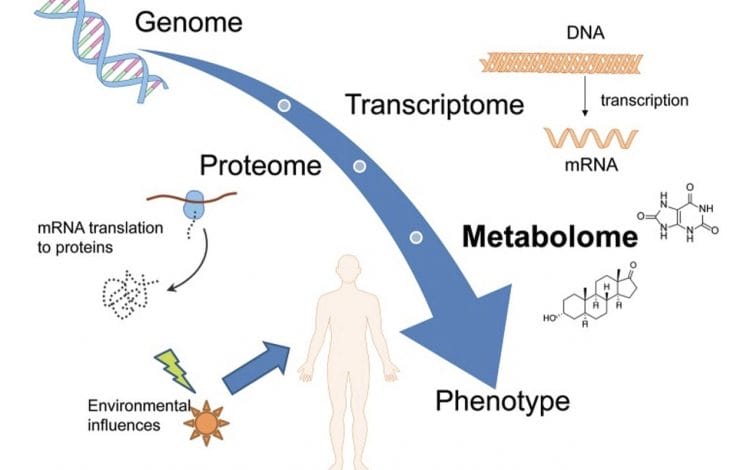
What is Metabolomics, anyway?
Metabolomics, a rapidly evolving field in scientific exploration, has emerged as a powerful tool for understanding the complex dynamics of biological systems [2]. By closely examining small molecules, including those generated by cellular processes and external sources, metabolomics goes beyond traditional boundaries, providing a comprehensive view of molecular landscapes. In the realm of biotechnology, this discipline is vital, as it dissects and quantifies metabolites in biological samples, offering insights into genetic, lifestyle, and environmental influences on metabolic processes. But the journey toward understanding metabolomics has been significantly accelerated by artificial intelligence (AI), promising to revolutionize research in the biotech sector.
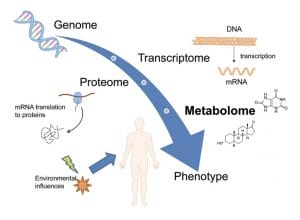
Why is it Important?
Metabolomics, particularly in the context of microbiome analysis, is at the forefront of answering critical questions in biotechnology. The growing interest in metabolomics, especially concerning the microbiome, underscores its pivotal role in uncovering the profound impact and functionality of this mysterious ecosystem. The gut microbiome, recognized as a crucial organ for maintaining health, has become a focus of inquiry, with implications reaching various aspects of human health, from conditions like irritable bowel disease (IBD) to unexpected associations with autism spectrum disorder and even cancer. Metabolomics provides invaluable insights into the metabolic intricacies of the symbiotic relationship between host organisms and their resident microbial communities. By understanding the metabolome’s role, we gain access to the molecular landscapes of microbial interactions, identifying disease biomarkers, and unravel host-microbiome dynamics, thus highlighting its significance in human health and disease.
![Figure 2 Schematic of a typical untargeted metabolomics workflow including data analysis, feature detection (peak picking), statistical evaluation and compound identification. [1]](https://zontal.io/wp-content/uploads/2024/03/untargeted_metabolomics_workflow--300x115.jpg)
How Can AI Help?
AI is not just advantageous but necessary in metabolomics research due to the challenges posed by the vast and complex nature of metabolomes. With its diverse set of tools and mathematical approaches, AI can process and analyze massive datasets inherent to metabolomic investigations. Its ability to uncover hidden patterns and distill complex data enables researchers to derive meaningful insights into the intricate metabolic landscapes governing biological systems. AI transcends traditional analytical boundaries, allowing computational systems to perform tasks that traditionally require human intelligence. From unsupervised learning methods like principal component analysis to supervised techniques like classification and prediction, AI offers a versatile arsenal tailored to the demands of metabolomic analysis. Advanced AI systems strategically combine multiple techniques to yield robust outcomes, unlocking mysteries within metabolomic datasets and propelling research toward new realms of discovery and innovation.
What’s Next?
In summary, the integration of AI into metabolomics research marks a new era of exploration and discovery, providing unprecedented insights into the metabolic landscapes of biological systems. Through a combination of supervised and unsupervised learning algorithms, AI empowers researchers to extract meaningful information from vast and complex datasets, leading to the identification of biomarkers, elucidation of metabolic pathways, and discovery of therapeutic targets. Looking ahead, the potential applications of AI in metabolomics are vast, with opportunities to refine existing methodologies, develop novel tools, and explore uncharted territories. In upcoming blog posts, we’ll delve deeper into the various AI methods employed in metabolomics, exploring their applications, limitations, and future directions. Join us as we navigate the frontiers of metabolomics research, guided by the endless possibilities offered by artificial intelligence.
Sources:
- Steuer AE, Brockbals L, Kraemer T. Metabolomic strategies in biomarker researchโnew approach for indirect identification of drug consumption and sample manipulation in clinical and forensic toxicology? Frontiers in Chemistry. 2019;7:319.
- Coler EA, Chen W, Melnik AV, Morton JT, Aksenov AA. Metabolomics in the era of artificial intelligence. Microbiota and Host. 2024;2(1).