DALL·E 2, Imagen, and Applications to Chemistry
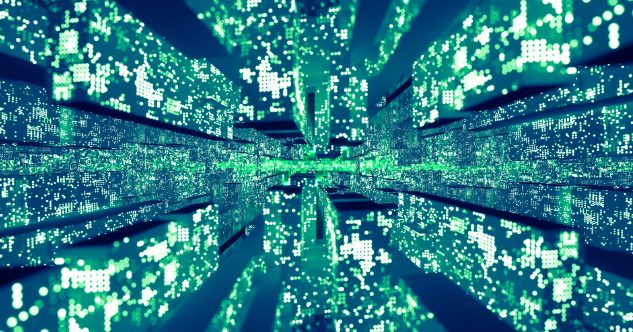
In the past two months, DALL·E 2 has taken over the internet. From Bart Simpson edited into Egyptian art to Donald Trump as the Lorax, text-to-image AI produces amazing results.
Caption: “Panda weaving a basket made of cyclohexane”, DALL·E 2
Are these an impressive-but-gimmicky party trick? Or can these innovations be harnessed for applications in scientific domains?
Many AI methods are developed in the laboratory before seeing practical adoption. This allows for measurable improvement of algorithms before real-life application. For example, reinforcement learning algorithms that were tuned on video games are now are used for robotics. Likewise, the Transformer architecture that was developed for text (admittedly a useful application in its own right) was recently adapted into AlphaFold, a model that has now folded every protein known to science.
The backbone of text-to-image AI is a newly popular type of neural networks called “diffusion models”. These models gradually transform an image of random pixels into a high-resolution image. The DALL·E 2 (from OpenAI) and Imagen (Google) models render photorealistic images from arbitrary text descriptions.
Caption: “Elephant toothpaste explosion with foam in the shape of an elephant”, DALL·E 2
So how can diffusion models help chemistry?
First, we should differentiate between conditional diffusion models and unconditional diffusion models. Unconditional diffusion models generate images randomly sampled from the distribution of the training data. On the other hand, conditional diffusion models modify that generated image based on some other type of input. In the case of DALL·E 2 and Imagen, this other input is text.
Diffusion models have some constraints. The output must have a fixed size – for example, diffusion models can generate images of fixed resolution, but they cannot generate variable-length sentences. Additionally, diffusion model outputs must be continuously-valued (though it is possible to generate continuously-valued embeddings of discretely-valued data). Finally, diffusion models are generative models, meaning that they are designed for sampling from distributions. So, it makes most sense to use them when there is an interesting distribution over an output variable, as opposed to a single correct value.
What chemistry prediction problems fall within these constraints? Two recent papers predicted molecular conformers using diffusion models conditioned on molecular SMILES strings. GeoDiff predicts molecular coordinates, and Torsional Diffusion predicts molecular torsion angles. Protein structure generation also fits within this framework, as another recent paper predicts protein atom coordinates conditioned on backbone constraints.
Caption: “Jedi uses a lightsaber to slice DNA in half”, DALL·E 2
Diffusion models have the benefits of the high-fidelity samples generated by generative adversarial networks (GANs) while maintaining a simpler architecture and being easier to train. Though chemistry applications are just beginning to emerge, diffusion models have yielded state-of-the-art accuracy in molecular conformer generation and offer a promising approach for other high-dimensional sampling problems.